An early warning system to predict clinical deterioration
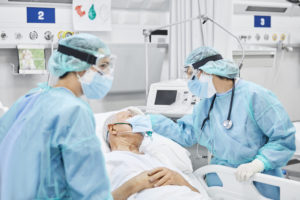
Increased access to Electronic Health Records (EHR) has motivated the development of data-driven systems that detect physiological derangement and secure a timely response. Commonly predicted adverse events such as mortality, unplanned ICU admission and cardiac arrest, have been extensively investigated by early warning systems (EWS), such as the National Early Warning Score (NEWS) that is currently recommended by the Royal College of Physicians in the UK. Typically, EWS systems assign a real-time alerting score to a set of vital sign measurements based on predicting risk to indicate the patient’s degree of illness.
However, physiological data recorded in EHRs are often sparse, noisy and incomplete, especially when collected in non-critical care wards. Missing information is often dealt with through complete-case analysis, the population mean imputation or carrying the most recent value forward. Such practices may impose bias and error and do not account for the uncertainty of the data.
Scientists at the University of Oxford have developed a novel approach to address these issues. They have developed an end-to-end framework which provides data pre-processing, patient-specific data modelling, and generates early warning scores to prevent adverse events which directly affect patient outcomes when undetected. Unlike the traditionally heuristic early warning scores, this system utilises deep learning with attention for enhanced patient risk stratification and improved model interpretability by clinicians. Each vital sign of each patient is initially modelled using a machine learning approach.
The modelled and regularly sampled vital signs are then classified according to this approach. The invention is useful towards predicting the risk of other adverse events and generally precision medicine, such as wearable health monitoring.
about this technology